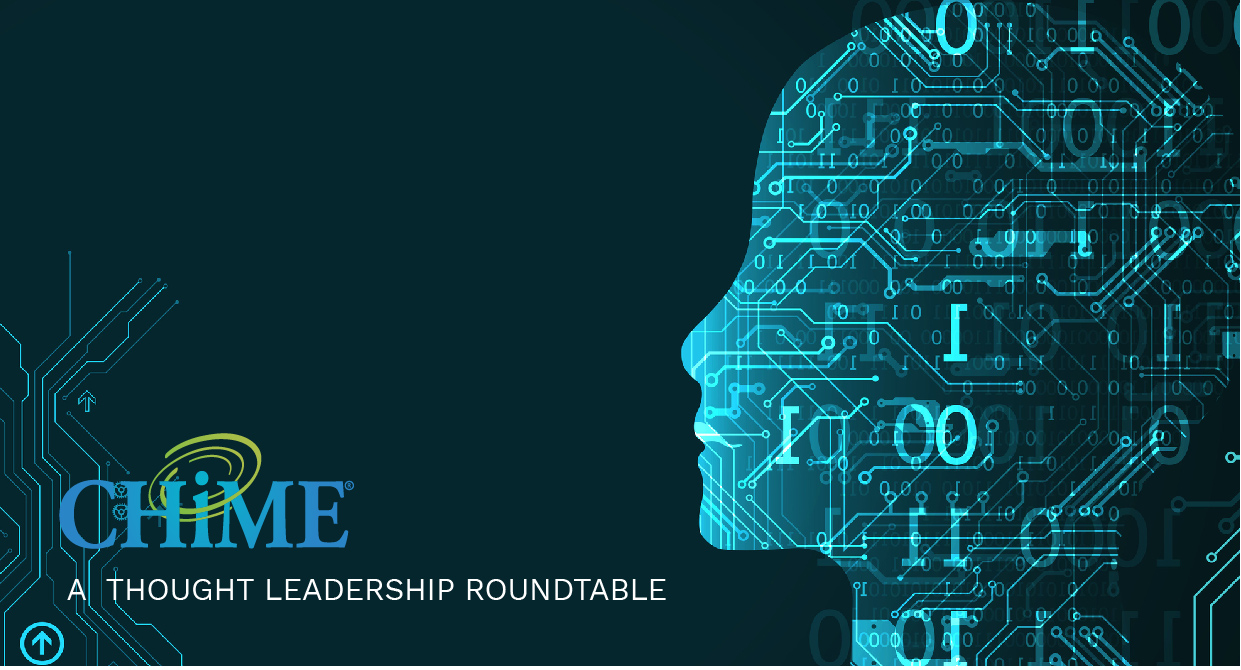
Ethical Use of Artificial Intelligence
Introduction
Cerner recently hosted a thought leadership roundtable featuring members of the College of Healthcare Information Management Executives (CHIME) to explore ethical issues related to artificial intelligence and machine learning. Chief information officers (CIOs), chief information and digital officers (CIDOs) and other digital health leaders discussed topics including algorithmic bias, measuring healthcare disparities across demographic groups, privacy, creating ethical review processes, federal regulation, and cross-industry collaboration. CHIME President and CEO Russell Branzell moderated the roundtable. Contributing to the discussion was Dick Flanigan, Senior Vice President, Cerner.
CHIME members participating:
- Kevin Bidtah, Chief Information Officer, Evergreen Health
- James Brady, VP, Information Security & Infrastructure/Operations, Chief Information Security Officer, Fairview Health Services
- Saad Chaudhry, Chief Information Officer, Luminis Health
- David Chou, Healthcare Technology Executive
- Adnan Hamid, Division Vice President & Chief Information Officer, Commonspirit Health, SoCal Region
- Paul Klehn, Chief Information Officer, Liberty Hospital
- Tressa Springmann, Senior Vice President, Chief Information and Digital Officer, Lifebridge Health
Summary
Artificial intelligence (AI) and machine learning (ML) hold the potential to revolutionize healthcare by leveraging data to improve quality, efficiency, and patient outcomes. Applications using AI and ML have flourished during the rapid digital transformation unleashed by the pandemic. Yet, case studies have shown that while AI and ML have the potential to transform patient care for the better, they also carry a risk of triggering unexpected consequences by amplifying disparities in health care. The roundtable gathered the perspectives of digital health leaders on how to realize the potential of AI while avoiding algorithmic bias and any other negative impacts on healthcare quality and access.
Current state of applied AI and ML in healthcare
Roundtable participants report that though the use of AI and ML in their organizations is rising, the technology is still in its infancy compared to the futuristic ideals often depicted in science fiction. IT leaders often have to clarify for their non-tech staff which types of AI and ML currently exist in their digital applications, in order to assuage fears caused by “intelligent robot takeover” stories and even predictions by tech industry leaders.
In healthcare, the most common types of AI in use in 2022 are based on robotic process-automation, which may include algorithms but does not include the kind of “learning” in which a machine becomes “smarter” without human assistance.
“Right now, our AI is making recommendations, not decisions,” said Tressa Springmann, SVP, CIDO, Lifebridge Health. “It recommends, but at the end of the day, it’s up to a human to click a button and go through with the approval.”
Despite the fact that the industry is still far from a world where thinking robots wield scalpels, AI has already proven that it carries more subtle but substantial risks. Even a human-supervised decision may trigger consequences from undetected bias buried in the algorithms.
AI software sometimes makes automated decisions that seem too small to be significant, but even those automations can have an outsized negative impact on patients. “In the assessment we’ve made in the nonclinical, administrative setting, there are many events when AI does take an action based on an automated decision,” said Dick Flanigan, SVP, Cerner. “The AI decides which bills go to collection and who gets certain care management resources, for instance. And those decisions can have negative consequences for certain patient groups.”
Ethical review in software acquisition
Leaders must often make buying decisions on software from third-party vendors based only on what those vendors claim about the embedded AI, without any additional means of evaluating the real-world impact of the AI. This buying position, often referred to as buying a “black box,” can present challenges to ethical review. “Most organizations buy commercial, off-the-shelf products,” said David Chou. “We are not developer shops: most of what we develop is on a third-party platform such as Microsoft, Google, or Amazon. Accordingly, we have to lean on ethical review by the vendors. And I don’t think we’re in a good position to really understand their algorithms because we are not developers.”
“In a community hospital, AI is not anything we develop internally,” said Paul Klehn, CIO, Liberty Hospital. “Instead, our AI is brought in by vendors who usually discuss their product’s use of AI based on revenue cycle, workflow, or strategies for market evaluation. They’re not presenting on community or social impacts.”
“I don’t know that I’ve yet seen an ethics section in a requirements document from a vendor,” said Jim Brady, VP and CISO for Fairview Health. “Generally speaking, it’s going to fall under privacy and security, but probably more on the privacy side where a lot of questions would be asked such as “How are you collecting the data? Is there informed consent? What about patient autonomy? That’s where some of the ethical questions might come from.”
“We may need to begin asking those questions now,” said Saad Chaudhry, CIO, Luminis Health. “Though currently, AI is often used in unsexy areas such as supply chain and voice activation, those kinds of applications do add up to a care continuum. So, there is an impact to patient care, even if it’s not direct.”
Adnan Hamid, DVP and CIO, Commonspirit Health, agreed. “We do often assume that commercial vendors have vetted out the potential impacts of their algorithms, but as we’re learning, our systems have bias in them because humans with biases are building them. We will need to address what will become an ethical issue down the road with AI.”
Liability questions raised by the absence of contractual language on negative impacts
As use of AI increases, leaders will need to consider liability and accountability for unforeseen negative impacts to individuals or groups.
“There is no language in our contracts right now that addresses accountability for undetected biases and any negative impacts they might cause,” said Klehn. “The most relevant language in most of our agreements is liability clauses that limit the vendor’s liability for any issues discovered with their products. So, if an organization is sued for any decisions made based on an AI-supported solution, the vendor will not be held accountable to the same level that the hospital system may be, especially in a care-providing situation.”
Preventing AI/ML tools from creating social disparities
Leaders acknowledge that the creation or exacerbation of social disparities by AI and ML algorithms is a possibility, and one that will need to be addressed through preventative planning.
One important principle is to always measure the stated goal of an AI tool in light of other potential goals and possible outcomes.
“If a CFO wants to implement a solution to increase revenue, there also has to be a discussion on the possible clinical outcomes,” said Brady. “We need to ask how this change will affect patients in our different cohorts. We need to have those conversations before we move forward.”
Constantly keeping multiple outcomes in conversation is part of a more holistic approach to care that can apply to the use of AI and ML as well as other aspects of healthcare.
“I think we’re shifting our approach to healthcare because we’re realizing that healthcare is relational, not transactional,” said Kevin Bidtah, CIO, Evergreen Health. “Having those checks and balances where we consider all potential impacts is part of the ongoing relational interaction between providers and patients.”
Others point out that while AI may have the potential to create disparities, the power of AI may be exactly what is needed to identify and solve those disparities.
“Biases have existed throughout human history and technology, and over time, data have helped us to identify biases,” said Klehn. “Why are we not using AI to help us identify biases?”
“That technology is on its way: there are people studying machine learning solutions today that will allow us to analyze what happened after an AI/ML implementation from a social-impact perspective,” said Flanigan. “So, the same technology that caused the problem may become the solution to that problem.”
Participants also point out that while there may be instances in which algorithmic bias in an application causes patients not to realize the full benefit they might have realized from removal of bias, the net effect of the application may still be beneficial.
“We’re implementing a very thin layer of AI over our bill payment that allows the system to look at the entire profile of a patient and offer payment methods that might benefit that patient,” Chaudhry said. “So, if something is missing from the algorithm and a patient doesn’t get every payment plan offer, but still receives more options than before the AI tool, then that patient still benefits even before the tool is totally perfected. That’s why it’s wise to take these technologies slowly: baby-stepping is important to allow providers to learn about those impacts and keep the technology beneficial overall as they improve it.”
Others agreed that adequate time is a crucial element of ethically implementing AI/ML-based solutions.
“Let’s say Company 1 creates an algorithm to predict something related to care,” Chou said. “Company 2 creates an algorithm for the same purpose. Now, whose algorithm do you trust? You’ve got to have time to process these options. It takes time to learn it, and it takes time to process a massive amount of data.”
“Instead of trying to hit it out of the park with AI, we’re going to do bunts and singles, and build that momentum to move on to the more complex cases,” Hamid said. “We need to be sure people are comfortable with AI and that they believe it’s actually helping us out.”
Collecting identity-based information
To combat disparities in digital health and keep AI from triggering any additional disparities, organizations will have to collect identity-based information about patients. Without collecting particulars about race, age, sex, ethnicity, socioeconomic status, sexual orientation and gender identity (SOGI), data analysts would have no way to track adverse impacts to discrete patient groups. At the same time, collecting that information might increase the potential for harm if such sensitive data is not managed and deployed well.
Roundtable participants understand that need for great care with sensitive information.
“Organizations are going to win or lose on how they commoditize data at scale,” said Springmann. “Even in local healthcare ecosystems, the organizations that better harness their data to help them understand their community get better outcomes. Whether you’re on the selling, buying, or provider end, the winners are going to be people who are thoughtful in managing the data.”
“We need to identify the different kinds of populations that either are missing or not represented appropriately, and make sure that’s factored in,” said Brady. “Otherwise, we will have a lot of data, but it’s not going to be complete.”
Leaders point out that the understanding of community and individual needs gained through the collection of sensitive data is essential to providing for those human needs.
“With patient experience in mind, we’re asking, ‘how are we positioning ourselves for people to be able to access care and feel comfortable and welcomed into our health organization?’” said Bidtah. “For at least 7 years, we’ve been capturing sexual orientation and gender identity information, or SOGI. We’ve captured seven options for sexual orientation and six options for gender identity. And we do look at all the health outcome metrics based on that. We also have health initiatives for drug users, and we want folks who are seeking that care to come to us, wherever they are in that process—we want them engaged in care. So, how do we shape the way we’re presenting themselves, and how do we shape their experience with us?”
Issues of diversity, equity and patient communication also must use these types of data to track progress.
“Our year-by-year vision through 2030 is strong in diversity, equity and inclusion goals that need to track this data,” said Chaudhry. “One goal, for example, is to expand the generational scope of the workforce. And there’s another initiative going on that is focused on equity and access from an ethnicity perspective. So that means we need to determine what signage needs to be in which languages (beyond English) for which campus. And to do that, you need the data.”
As leaders consider how to gather data to assess diverse patient populations and their needs, another important consideration is that one institution may not have access to a complete portrait of a local community.
“Most healthcare organizations are not the only player in town,” Flanigan said. “In really large cities, they aren’t even the only player in their part of town. And that means that while you can assess certain types of problems internally, there are also larger community issues that require you to have a much bigger world out there as your denominator.”
Sometimes, tracking patient identities with adequately specific data sets leads to surprising conclusions that defy conventional wisdom.
“When we looked at disparities in HIV/AIDS care and the health outcomes there, we found the inverse of what we expected,” said Bidtah. “We expected that the underserved minority populations would be doing worse, and they were actually doing better because they were more integrated into our system. They had case management, wraparound services, and housing assistance tied together, which gave them better opportunities to stay connected to care.”
Moving the conversation on AI/ML forward
The advent of revolutionary technology requires a response from organizations and from the industry at large as healthcare leaders attempt to maximize the benefits of AI/ML and avert any potential negative outcomes. Leaders discussed several collaborative approaches that would help the industry, beginning with conversations that engage all the stakeholders of a provider organization.
“The conversation on AI and ethics needs to be led by the clinical and business side,” said Hamid. “If it becomes something that IT is driving, it won’t be as well-received because of the risk that it will come across as just another IT tool.”
Others see the role of the CIO in the AI/ethics conversation as part of the increasing involvement of CIOs in that operational side of a provider organization. “The CIO of yesterday has to be the digital officer of the future, which is ingrained in operations,” Chaudhry said. “That conversation does have to be led from the operational side.”
Cooperation between providers and vendors will also be crucial to the effort to review AI/ML tools for ethical impact.
“There’s got to be a joint venture,” said Chou. “There’s got to be some skin in the game from both the client and the vendor to co-innovate. I don’t think that has ever happened, but there must be more incentive to get this moving from the vendor space. They create the black box, and we become buyers of it. But we don’t look deeply into how the products develop all the AI. So, any co-development would be a great start.”
Chou also points to the need for teamwork and data sharing between healthcare organizations. When healthcare providers in the same region don’t share information that could benefit their communities because those organizations see their situation as competitive, patients lose.
“They’re not co-innovating with their data,” said Chou. “And that also puts a vendor at risk for developing their product, because they may not get all the innovation and data that can be brought together from the client side.”
Three steps to take now to review AI/ML initiatives
If forward-thinking leaders wish to ensure that AI/ML technology remains ethical and liability-free, they will have to act now to stay ahead of the rising tsunami of innovation in the industry.
Flanigan recommends three steps that leaders can take now to maximize the chances that AI will bring only good and no harm to the people served by the healthcare ecosystem.
1) Comprehensive assessment
Make a full inventory of where AI and ML algorithms are currently used by the organization, including instances when those algorithms are embedded in third-party software.
2) Analyze outcomes
Conduct ongoing monitoring and testing for bias and unintended adverse consequences.
3) Develop a process to address negative findings
Consider the need for an independent review board, a patient safety organization, or another neutral party to evaluate findings and address necessary remedies.
Industry-wide efforts needed
Roundtable participants agree that collaborating across organizational boundaries will be enormously beneficial to help the industry toward ethical use of AI.
“One of the things we struggle with is finding a common vernacular,” said Springmann. “Coming up with a common language around AI and robotic process automation to demystify these discussions would be a basic place to start. My recommendation would be to set a foundation by creating some educational resources, so that as peers, we have a common vernacular.”
Klehn added, “As CHIME members, we have to understand that though our primary audience is CIOs, the audiences they support are quite diverse and vary widely in their understanding of AI. So, we would need to put together education and professional development to address audiences at different levels.”
“I think we can put together the framework to educate people, but let’s also get the other executives who are leading the organizations to be thinking along with us and moving forward with us,” said Chou. “That way, everyone is working together on solutions, instead of the perception that IT is bringing up a problem for others to solve.”
“Getting to common vernacular and sharing best practices could help out our standalone community hospitals and rural hospitals that have fewer resources,” said Hamid. “Sometimes, those hospitals are ahead of the game in using AI because they have to automate to compensate for missing resources. Any tools and documents CHIME could provide for them would be extremely helpful.”
“Certification at some level could also encourage these kinds of progress, whether it’s the shared vernacular or the framework,” said Chaudhry. “For example, the CHIME Digital Health Most Wired Survey allows most CIOs to understand what it means if one of their peers tells them, ‘Our organization is a Most Wired Level 8, or Level 9.’ Creating a certification in this area might help us speak the same language about what we’re doing in this area for AI and ML.”
Finally, leaders pointed to the need for expert-led discourse to shape the yet-to-be-written federal regulation that will eventually emerge to guide AI and ML.
CHIME has already submitted recommendations to the U.S. Department of Health and Human Services that contained suggestions on ethical considerations related to AI. This policy advocacy must continue through CHIME’s Policy Steering Committee and other industry advocates who bring crucial frontline experience to the regulatory discussion.
Conclusion
As the use of AI/ML increases across the healthcare industry, digital health leaders must consider how to prevent advanced technologies from creating unintended social disparities through algorithmic bias. Many AI algorithms are embedded in third-party software purchased by a healthcare provider as a “black box,” so the provider operates without first-hand knowledge of the algorithms inside that black box. Leaders must work with vendors to consider ethical issues and liability for any unintended negative consequences of the AI tools.
Additionally, healthcare organizations need to develop ethically conscious processes to assess, analyze, and review their own AI/ML-driven outcomes for social impact, and then consider remedies for negative impacts. Collecting identity-based information from consumers is a risk-laden but crucial part of the process of identifying social impact on specific patient groups; therefore, implementing safe, private technology to hold that data is imperative.
Federal regulation will also be a part of the new landscape after the AI/ML revolution, and policy advocacy from industry experts must help ensure that any regulatory activity is informed by real experience from the front lines.
Collaboration across the industry will be key to the ethical use of AI/ML, and groups like CHIME have a key role to play in bringing together digital health leaders for education and resource-sharing to start industrywide dialogue.
This thought leadership roundtable article was written by Rosslyn Elliott, CHIME Editor, and brought to you by Cerner.
RETURN TO CHIME MEDIA