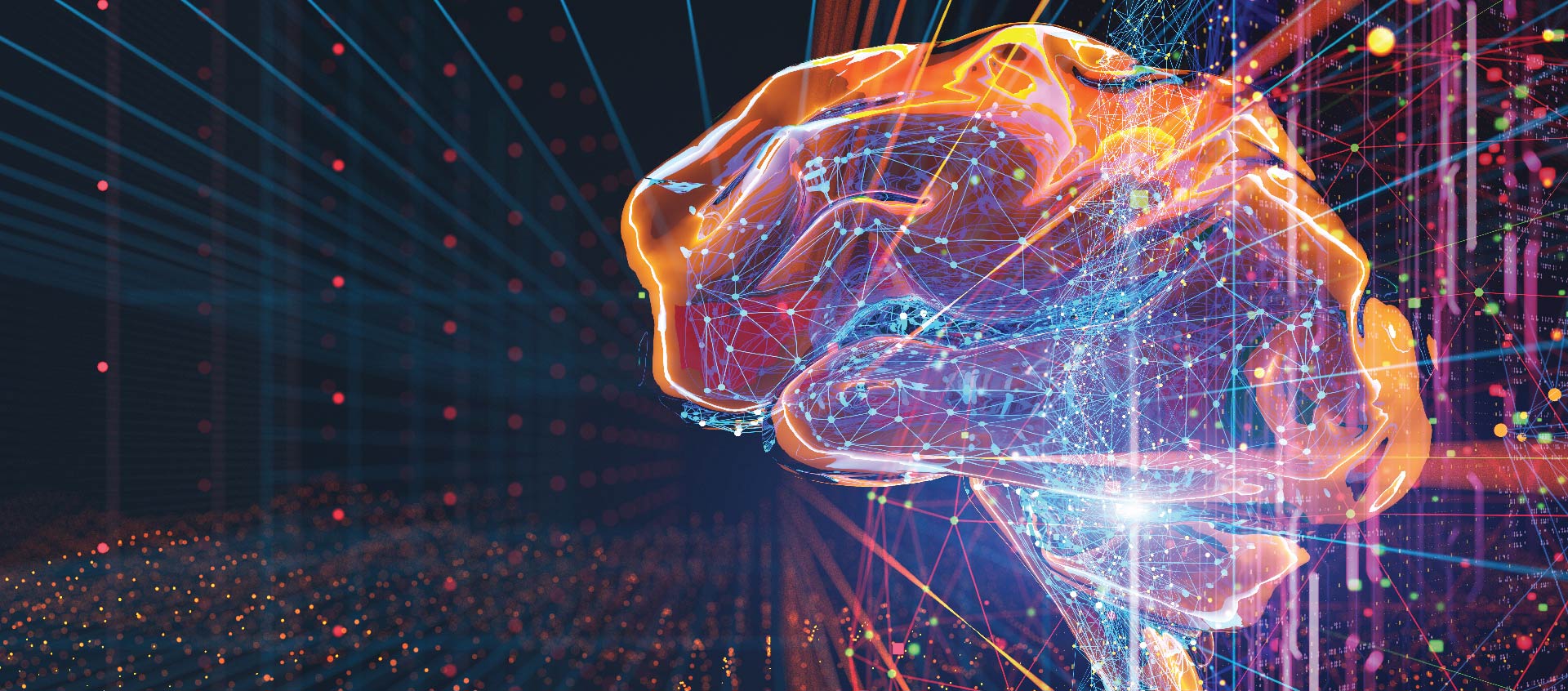
Machine Learning in Healthcare: A Thought Leadership Roundtable
Introduction
Hewlett Packard Enterprise (HPE) recently hosted a thought leadership roundtable featuring six members of the College of Healthcare Information Management Executives (CHIME) to discuss machine learning (ML) in healthcare organizations. Chief information officers (CIOs) and chief digital and information officers (CDIOs) discussed best practices and how to realize the full potential of ML to improve patient outcomes and organizational efficiency. CHIME President and CEO Russell Branzell moderated the roundtable, and HPE’s Executive Healthcare Strategist Steve Shim and Distinguished Technologist Reza Bacchus contributed to the discussion.
CHIME members participating were:
- Lisa Stump, Senior Vice President, Chief Information Officer, Yale New Haven Health System and the Yale School of Medicine
- Donna Roach, Chief Information Officer, University of Utah Hospital and Clinics
- Mike Minear, Senior Vice President, Chief Information Officer, Lehigh Valley Health Network
- Nassar Nizami, Executive Vice President, Chief Information and Digital Officer, Thomas Jefferson University and Jefferson Health
- Cletis Earle, Senior Vice President and Chief Information Officer, Penn State Health and Penn State College of Medicine
- Craig Richardville, Senior Vice President, Chief Information and Digital Officer, SCL Health
Summary
ML is a subset of artificial intelligence (AI) that focuses on using algorithms against large data sets. ML can “learn” from a data set to improve its own analysis without the need for additional programmer input of new analytical rules. As the healthcare industry searches for solutions to analyze and apply the vast amount of data collected through electronic health records (EHRs), ML is a natural choice to successfully deploy that data. The power of ML can transform the quality and accuracy of healthcare, but first, industry leaders must address the challenges that accompany implementation and oversight.
Current state of applied ML in healthcare
The application of AI and ML in healthcare settings is in its early stages, but definitely on the rise, as revealed in the CHIME Digital Health Most Wired Survey of 2020. In order to discuss the state of applied ML at organizations that are early adopters of emerging technologies, roundtable participants were drawn from large healthcare systems, many with affiliated academic institutions.
These organizations tend to follow a similar pattern in the structure of their data and analytics teams. On the clinical or applied side, these healthcare systems have teams that often include one or more data scientists and report to the CIO. On the research side, there are even more data scientists and researchers working on ML-based initiatives, and CIOs are currently working to integrate the work of the researchers with the clinical side. The enterprise data warehouse (EDW) can serve as a shared asset between a health system and a school of medicine, as it does at Yale New Haven Health System. There are five analytics teams at Lehigh Valley Health, each dedicated to a different application: enterprise analytics, two informatics teams focused on medical/nursing applications, consumer analytics, and popular health topics. Some organizations have focused primarily on operational uses for ML such as house census or risk of ED admissions, while others have chosen diagnostics and advanced clinical decision support as their chief ML target.
‘The data belongs to the patient’: data-sharing in the deployment of ML
While many organizations are eager to realize the potential benefits of ML, some common challenges hinder widespread implementation. The most significant barrier is the lack of data-sharing across health systems. Increasing interoperability would allow organizations to share information by creating architectures that allow for frictionless data-sharing.
While discussions of interoperability often focus on technology challenges, a crucial element of data-sharing is often overlooked.
“It’s important to reinforce that the data belong to the individual patient, not to us as organizations,” said Lisa Stump, CIO for Yale New Haven Health System and the Yale School of Medicine. “The decision to share and use the data rests with the patient, and we have an obligation to ensure they understand where their data are being used.”
Other participants agreed and added that the patients’ ownership of their data presents exciting possibilities for the long-term problem of organizational resistance to data-sharing.
“I think the breakthrough will be when patients are truly owners of the data, and they can share data with anyone,” said Nassar Nizami, CIDO at Jefferson Health and Thomas Jefferson University. Nizami described a model such as Apple’s, in which patients can share data from their EMR with the click of a button. “And with the help of the technology we have, all patients must be able to share it with whoever that other party is, whether it’s a startup or government.” Patient ownership of data will also help with another issue that Nizami sees on the horizon: the growing importance of data that non-healthcare organizations will hold.
If all data-holders are required to make a patient’s data available in a standardized, usable format, transformation from ML will be swift and revolutionary in effect. Enabling patients to control their own data would also overcome the longtime institutional resistance of many health organizations to information-sharing that currently frustrates attempts to raise ML to a new level.
Collaboration on data-sharing between health systems for community benefit
“Access to data is not the differentiator, in that all data should be available to every health system,” said Craig Richardville, CIDO for SCL Health. “However, how we actually use the data, that is the differentiator and how we can distinguish ourselves and provide leadership for each other.” Richardville and other participants agreed that competition between health systems needed to be balanced with increased collaboration for the good of the industry at large and most importantly, for patients. Cletis Earle, CIO of Penn State Health and Penn State College of Medicine, described his desire to further his work with regional CIOs to level the playing field of information sharing. “We are as good as the information that we have accessible to us, so that we can give it to our providers to get the best care for our patients.”
“Yes, we’re competitors, but we should be working on things that benefit our entire community,” said Donna Roach, CIO of University of Utah Hospital and Clinics. Roach pointed out that more unified action across the boundaries of health systems could reap benefits in collective negotiations with vendors and united advocacy efforts in state legislatures.
In some cases, data-sharing must cross tech barriers that are even greater than software incompatibility, and yet those cases are crucial to health equity. Mike Minear, CIO of Lehigh Valley Health Network, described the problems and opportunities in acquiring data while working with public health partners such as local educational organizations, criminal justice, and municipal public health agencies. “When we talk with these partners, they have comparatively little technology capability,” Minear said. “Many community organizations still have some paper-based processes and a patchwork of software applications. As care providers focus more on social determinants of health, partnerships with non-clinical organizations will be critical. We are now hosting public health agencies with our EHR and sharing data to better understand and serve patients who have social issues that impact their health status. Health providers must expand their view and analytics beyond the walls of hospitals or clinics to provide optimal care for the patients we serve.”
Interoperability and innovation from the tech industry
Innovation is very important to the development of ML and its potential to transform healthcare. As roundtable participants noted, many brilliant innovators working in technology are in need of data to develop and deploy their products, but they do not have access to the data under today’s siloed system. If patients control their own data, the innovation sector could also benefit greatly from the consequent removal of organizational resistance to data-sharing.
Currently, innovators are handicapped by a lack of understanding of the kind of architectural thinking that must accompany their innovations. The most advanced health systems are planning long term for maximum compatibility across tech systems. “Common data models and mapping of the data are critical,” said Lisa Stump. “We’ve invested time, energy, and effort in building a computational health platform, adopting the OMOP Common Data Model to ensure that we can both ingest and aggregate external sources, but also potentially export the data. That could be useful to data innovation across the industry.” Stump added that when she asks startups how they will approach the data model and underlying architecture, she frequently does not hear a fully developed strategy in response.
Local, central, or federated learning: improving patient privacy and data efficiency
Patient privacy is an ongoing concern in data-sharing at all levels across the industry.
One strategy to prevent privacy risk and simultaneously improve the ease of data-sharing is federated learning, according to Reza Bacchus, Distinguished Technologist at HPE. Federated learning shares only the learnings from data between systems rather than the data itself. Sharing learnings carries an enormous advantage in efficiency. For example, if systems have to share hundreds of thousands of x-rays, the bandwidth required for all the pixels of data is very wide. By contrast, through federated learning, those systems can share only learnings, so there is much less need for bandwidth or storage in the centralized data location. Health systems can employ technologies such as blockchain for additional security to keep rogues out of the data. Having patient data remain within the controlled environment of the covered entity best ensures privacy compliance and eliminates arduous procedures for transferring data.
Conclusion
As CHIME CEO Russell Branzell pointed out, other countries around the world have made great strides in data-sharing with de-identified data, so their health systems can now leverage ML for unprecedented levels of operational efficiency and diagnostic capability. Healthcare systems in the USA need to identify a path forward to achieve similar quality outcomes.
By advocating for the right of patients to control their own data and supporting universal standards that require that data to be shareable, healthcare organizations can overcome most of the current challenges that stand in the way of a great technological leap forward with the power of ML. If healthcare leaders can come together to create the necessary conditions for implementation, the potential of ML applications is unlimited to solve many healthcare efficiency issues, alleviate the burden on clinicians, and improve outcomes for patients.
This thought leadership roundtable was written by Rosslyn Elliott, CHIME Editor, and brought to you by Hewlett Packard Enterprise (HPE).
RETURN TO CHIME MEDIA